Accounting for the Changing Role of Family Income in Determining College Entry
Testo completo
(2)
(3) EUROPEAN UNIVERSITY INSTITUTE. DEPARTMENT OF ECONOMICS. Accounting for the Changing Role of Family Income in Determining College Entry CHRISTOPH WINTER. EUI Working Paper ECO 2007/49.
(4) This text may be downloaded for personal research purposes only. Any additional reproduction for other purposes, whether in hard copy or electronically, requires the consent of the author(s), editor(s). If cited or quoted, reference should be made to the full name of the author(s), editor(s), the title, the working paper or other series, the year, and the publisher. The author(s)/editor(s) should inform the Economics Department of the EUI if the paper is to be published elsewhere, and should also assume responsibility for any consequent obligation(s). ISSN 1725-6704. © 2007 Christoph Winter Printed in Italy European University Institute Badia Fiesolana I – 50014 San Domenico di Fiesole (FI) Italy http://www.eui.eu/ http://cadmus.eui.eu/.
(5) Accounting for the Changing Role of Family Income in Determining College Entry Christoph Winter∗ European University Institute Department of Economics JOB MARKET PAPER October 31, 2007. Abstract Assessing the importance of borrowing constraints for college entry is key for education policy analysis in the U.S. economy. I present a computable dynamic general equilibrium model with overlapping generations and incomplete markets that allows me to measure the fraction of households constrained in their college entry decision. College education is financed by family transfers and public subsidies, where transfers are generated through altruism on part of the parents. Parents face a trade-off between making transfers to their children and own savings. Ceteris paribus, parents who expect lower future earnings transfer less and save more. Data from the 1986 Survey of Consumer Finances give support to this mechanism. I show that this trade-off leads to substantially higher estimates of the fraction of constrained households compared to the results in the empirical literature (18 instead of 8 percent). The model also predicts that an increment in parents’ earnings uncertainty decreases their willingness to provide transfers. In combination with rising returns to education, which makes college going more attractive, this boosts the number of constrained youths and explains why family income has become more important for college access over the last decades in the U.S. economy. Keywords: College Enrolment, Borrowing Constraints,Parental Transfers, Household Savings, Dynamic General Equilibrium Models JEL classification: I20, I22, D58, D91 ∗. A previous version of this paper was circulated under the title ”Explaining Earnings Persistence: Does College Education Matter?”. I would like to thank Morten Ravn and Salvador Ortigueira for their advice. I also benefited from comments of Aurora Ascione, Judith Ay, Renato Faccini, Ken Judd, Dirk Krueger, John Knowles, Ramon Marimon, Joana Pereira, Franck Portier, V´ıctor R´ıos-Rull, David Scherrer and Ken Wolpin as well as participants at the SMYE 2007, the European Macro Workshop 2007, the X. Summer School of the Fundaci´ on Urrutia Elejalde and the ECINEQ 2007. A first draft of this paper was written while I was visiting UPenn. Address: Via della Piazzuola 43, I-50133 Firenze, Italy. E-mail: christoph.winter@eui.eu.
(6) 1. Introduction. In the United States, the wage premium of college graduates relative to high school graduates increased by around 30 percent between 1980 and 2000 (Katz and Autor (1999)). This period was also characterized by a dramatic rise of college tuition fees and an amplification of the within-group earnings inequality. College participation rates stagnated, while the enrolment gaps between students from different family income groups widened (Ellwood and Kane (2000), Carneiro and Heckman (2003) and Kane (2006)). This suggests that financial constraints prevent a larger share of low-income households from sending their children to college, leading to a sluggish adjustment of college participation despite the surge in the college premium Kane (2006). Whether this is true or not is subject to an ongoing debate in the empirical literature. Carneiro and Heckman (2002) and Carneiro and Heckman (2003) argue that short-term cash constraints around college age are binding only for a small fraction of households. They find that long-term factors (family background variables) which affect pre-college education can account for the main part of enrolment gaps by income. However, it is not clear to what extend long-term factors can account for the widening of the enrolment gaps observed over time. Belley and Lochner (2007) document that the impact of family income on college attendance rates increased dramatically between 1980 and 2000, even after controlling for family background. They also document that the enrolment patterns observable in the data are at variance with a simple model of college attendance, even if they allow for borrowing constraints. Hence, there remains considerable disagreement about the role of borrowing constraints (Kane (2006)). In this paper, I want to shed further light on the role of financial constraints. In particular, I address the following two questions: 1. Are borrowing constraints quantitatively important in determining college entry? 2. As the economic environment has changed in the U.S. over the course of the last decades, have borrowing constraints become more limiting? I answer these questions with the help of a computable overlapping generation model that endogenizes the college enrolment decisions. Borrowing by young households for college education is not permitted; they thus have to relay on parental transfers and public support in the form of subsidies in order to cover college expenses. This allows me to measure the fraction of adolescents that would like to attend college but cannot do so because of market imperfections. I derive the distribution of parental transfers endogenously in my model; this enables me to study the impact of the recent changes in the economic environment on college attendance and on borrowing constraints. I show that the enrolment gaps produced by the model narrow considerably once I control for so-called long-term factors. Thus, the findings are consistent with Carneiro and Heckman (2002). Yet, I find that a substantial fraction (18 percent) of all households are borrowing constrained. This shows that even when enrolment gaps are narrow, borrowing constraints may affect a large part of the population. The results thus help to resolve the disagreement in the empirical literature with respect to the quantitative importance of borrowing constraints. 1.
(7) I then examine how the economy behaves if I increase the college premium, the tuition fees and the earnings inequality to values observable in the U.S. economy around 2000. I find that that the model replicates the college enrolment patterns presented in Belley and Lochner (2007) very well. In particular, the model predicts (i) a slight increase in the number of college graduates, (ii) a substantial increment in the impact of family income on the college enrolment of young households, and a (iii) stable ability-enrolment pattern. The model predicts that the fraction of constrained households rose sharply from 18 percent to 40 percent between 1980 and 2000. The results thus show that all enrolment patterns can be explained within the same framework, and that these patterns are consistent with an increase in the number of constrained households. Despite the sharp rise in the number of households affected, the model implies that the correlation of educational attainment across generations actually decreased. This is perhaps surprising, as the literature in general assumes that tighter borrowing constraints lead to a higher persistence of education across generations. Ellwood and Kane (2000) as well as Belley and Lochner (2007) document that the correlation between parental education and college enrolment of the child has become weaker over the course of the last decades. Understanding the behavior and the determinants of parental transfers is crucial for my results. The model endogenously accounts for the initial distribution of wealth of young households by assuming that altruistic parents provide transfers to their children.1 More precisely, I assume that old households (’parents’) are altruistic and incorporate the utility of their descendants (’young households’) in their maximization problem. I follow Laitner (2001) and allow for imperfect altruism; parents may weight their children’s utility less than their own utility. In the model, I distinguish between two different levels of human capital (’college education’ and ’high school’) and endogenize college choice. Parental transfers can be used to finance college education, which is assumed to be costly. I also allow for idiosyncratic labor income shocks, which enables me to analyze the effects of the rise of the within-group inequality that has been documented for the U.S. (Krueger and Perri (2006)). Incorporating inequality within generations and education levels also allows me to distinguish different ability levels. I solve the model numerically and calibrate the parameters such that key features of the U.S. economy are matched. I then compare two different steady-state equilibria in order to evaluate changes over time. Altruism implies that there exists a trade-off for parents between investing in their own future or in the future of their children. This means that even children from rich families 1 Gallipoli et al. (2006), and Ionescu (2007), among others, assume that the initial distribution of wealth is exogenous for young households. Hanushek et al. (2004) assume that transfers are generated by assuming a ’joy-of-giving’ motive, which implies that transfers depend only on parental wealth but not on the economic situation of the child. This is at odds with empirical evidence showing that inter-vivos transfers are negatively correlated with the child’s permanent income (Cox (1990)). Restuccia and Urrutia (2004) and Cunha (2005) use an altruistic framework to analyze the parental trade-off between human capital investments at different stages of life. Because their focus is on early education, they model parents only up the age when their children enter college. I show that all stages of the parental life cycle are important in order to understand transfer behavior.. 2.
(8) can be constrained in their college decision because their parents are not willing to provide enough resources. Indeed, I show that the transfer flows generated by the model imply that parents consider their offspring’s utility by 30 percent less than their own utility. This worsens the position of the child and pushes the number of constrained households up. In fact, I show that the parental trade-off explains why borrowing constraints are binding for a substantial fraction of the population, even though enrolment gaps across different income groups appear to be narrow, after controlling for long-term factors. The model also allows me to study how the trade-off between savings and transfers evolves over time. I show that the interplay between the rise in the between-group and in the within-group inequality explains why borrowing constraints became more limiting over time. The rise in the college premium (between-group inequality) makes college investment more profitable, even for low-ability youths. However, their parents accumulate additional savings in order to self-insure against the increase in the within-group inequality, which I model as an increment in the variance of earnings shocks. This implies that accumulating more savings is preferred over investing in college education for parents with low-ability children. It follows from the model that the increase in the enrolment gap is more pronounced for low-ability students. This is exactly what one observes in the data as well (Belley and Lochner (2007)). Since earnings of high school graduates fluctuate more than the earnings of college graduates (Hubbard et al. (1995)) and earnings account for a bigger fraction of total income for high school graduates, this channel also helps to explain the degree of intergenerational persistence of educational attainment. In my framework, children of college graduated parents are – all other things equal – up to 5 percent more likely to enter college, because their parents need to provide less savings for their own future. Using transfers and savings data from the 1986 Survey of Consumer Finances (SCF), I find empirical support for this key prediction of the model: high school graduates save significantly more than college graduates during the last 20 years before retirement if one controls for wealth and income. In turn, college graduates in that age group provide significantly more transfers. The remainder of the paper is structured as follows. I present the model in section 2. Section 3 introduces the equilibrium definition, while the calibration is explained in Section 4. We discuss our results in Section 5. Finally, Section 6 concludes.. 2. Model. I consider a life cycle economy with altruistic parents. As in Laitner (2001), altruism may be imperfect. Parents provide transfers to their children. They face constraints on their resources: all credit markets are closed, implying that they can neither borrow against their own future income nor against the future income of their descendants. I allow for idiosyncratic productivity shocks during working life. Moreover, I endogenize college choice by assuming that parental transfers can be used to pay for college education. These assumptions allow me to study the effects of an endogenously generated initial distribution of assets on college enrolment, and to analyze the determinants of the initial asset distribution in a realistic life cycle setting. 3.
(9) 2.1. The Life Cycle of a Household. There is a continuum of agents with total measure one. I assume that the size of the population is constant over time. Let j denote the age of an agent, j ∈ J = {1, 2, ..., J max }. Agents enter the economy when they turn 23 (model period j = 1). Before this age, they belong to their parent household and depend on its economic decisions. During the first 40 years of their ’economic’ life, agents work. This implies that the agents work up to age 62 (model period J work = 40). Retirement takes place at the age of 63 (j = 41), which is mandatory. When agents turn 53 (j = 31), their children of age 23 form their own household. This implies a generational age gap of 30 years. It is assumed that there is one child household for each parent household. Agents face a declining survival probability after their children leave home. Terminal age is 83 (J max = 60). Since annuity markets are closed by assumption, agents may leave some wealth upon the event of death. The remaining wealth of a deceased parent household is passed on to its child household.. 2.2. Transfers. At age 53, a parent’s household child becomes independent and forms its own household. Gale and Scholz (1994) report that the mean age of givers is 55 years in the 1983-1986 Survey of Consumer Finances. I assume that transfers are generated by one-sided altruism, that is, parents care about the lifetime well-being of their mature children, but not the other way round. I abstract from strategic interaction and assume that parents provide part of their own wealth as an initial endowment at the beginning of the economic life of the child household. Part of this endowment (or all of it) can be in form of investment in human capital. It is important to notice that the assumption of altruism implies that parents will combine education investment and financial transfers in such a way that the child’s lifetime utility is maximized given the total amount of wealth that parents wish to pass on to their descendants. Put differently, children may not agree with their parents on the total amount which is being transferred, but certainly on the mix between human capital investment and financial transfers.2 The assumptions regarding the life cycle and the transfer behavior are summarized in Figure 1.. 2.3. Labor Income Process. During each of the 40 periods of their working life, agents supply one unit of labor inelastically. The productivity of this labor unit of an j-year old agent is measured by εej η j,e , J w where εej j=1 is a deterministic age profile of average labor productivity of an agent with education level e: e ∈ E = {highschool(hs), college(col)} (1) 2 In the following, I will use the terms ’financial transfers’, ’inter vivos transfers’ and ’inter vivos transfers’ as labels for capital transfers which take place during lifetime of both donor and recipient.. 4.
(10) Retirement. Working life 23. • Work, consume, save. 53. • Idiosyncratic productivity risk. 63 • consume. 83. • mortality risk • bequests. • investment in offspring’s education. Agents characterized by • assets (from parents). • inter-vivos transfers of wealth. • education & product.. Figure 1: Life cycle and Generation Structure For retired agents, εej = 0. η j,e describes the stochastic labor productivity status of a j-year old agent with education level e. Given the level of education e, I assume that the labor productivity process is identical and independent across agents (no aggregate productivity shocks) and that it follows a finitestate Markov process with stationary transition probabilities over time. More specifically, Q(η hs , N hs ) = Pr(η j+1,hs ∈ N hs |η j,hs = η hs ) (2) for high-school graduates. N hs = η1hs , η2hs , ..., ηnhs is the set of possible realizations of the productivity shock η hs . Similarly, I express the stochastic labor productivity process for college graudates as (3) Q(η col , N col ) = Pr(η j+1,col ∈ N col |η j,col = η col ) with N col = η1col , η2col , ..., ηncol . I assume that children of college graduates have - on average - productivity levels above average, while high school graduates draw shocks that are below average. Carneiro et al. (2006) show that maternal education has a strong positive impact on children’s cognitive achievement. I interpret the initial draw as a proxy for ability during adolescence, that is, ability before college education or labor market entry occurs. In particular, I assume that the probability to dropout from college decreases with the level of the initial productivity shock. Consistent with empirical evidence regarding the intergenerational correlation of schooling, this and the the fact that the productivity in the first period of working life depends on parental education, implies that college education is positively correlated across 5.
(11) generations. The parental education level influences only the initial draw of the productivity shock: From the second period onwards, the shocks evolve according to their respective stochastic process. More specifically, I assume that the initial shock is governed by the following transition matrices:. 2.4. Qinitial,hs (i, i ∈ I = {1, 2, ..., n}) = Pr(i, i ∈ {1, 2, ..., n} |η 30,hs = η hs ). (4). Qinitial,col (i, i ∈ I = {1, 2, ..., n}) = Pr(i, i ∈ {1, 2, ..., n} |η 30,col = η col ). (5). Investment in Education and Borrowing Constraints. I distinguish between two levels of education, high school and college.3 Upon entering the economy, all households possess a high school degree. They (or their parents) decide on investing in college education, before any other economic action is taken. Investment in college education takes place at the beginning of the lifetime. College education requires large investments that are risky and lumpy. It is risky because there is a certain probability that the child drops out. In addition, the earnings stream is stochastic which increases the uncertainty. Dropout rates are high in the U.S., as well as in other OECD countries (see Akyol and Athreya (2005)). Consistent with evidence from the empirical literature, see e.g. Stinebrickner and Stinebrickner (2007), I assume that children with lower levels of ability are more likely to drop out. Since dropout rates are higher during the first years of the college studies (when returns to college education are low), dropouts face the same earnings process as high school graduates. Consequently, only students who actually graduate from college enjoy higher mean earnings during their working life. This implies that college education is an indivisible and lumpy investment.4 Transfers and savings cannot be negative; parents are thus required to finance their children’s college education out of their own resources.. 2.5. Taxes and Social Security Benefits. During working life, households pay a proportional tax on their labor income. All households also pay a proportional tax on their capital income. Tax revenue from labor income and capital income taxation is used by an infinitely lived government in order to finance pension benefits pen. I assume that pensions are independent of the employment history of a retiree.. 3. The Households’ Recursive Problem. I distinguish between young households (children) and parent households. I use a subscript y for young households and a subscript p for parental households. 3 The share of high school dropouts is small in the data, see Rodriguez et al. (2002) who measure a share of 17 percent in the 1998 SCF. 4 See Akyol and Athreya (2005) and the references cited therein.. 6.
(12) 3.1. Young households. When parents die, young households inherit the wealth of their parents. I assume that young households observe their parental wealth holdings. Therefore, I need to distinguish between child households with deceased parents and young households that are expecting to inherit. I make the following timing assumption: death takes place at the end of the period, after the consumption and savings decision has been made. Bequests are then distributed at the beginning of the next period. 3.1.1. Young households with deceased parents. Consider a household during working age (j ∈ J w = {1, ..., 30}) whose parent household is dead. At age j, this household consumes cy,d and has end-of-period wealth holdings of ay,d , where the subscript y, d indicates a young household with deceased parents. Given a discount factor β, a rate of return to capital r, a wage rate per efficiency unit of labor w, tax rates on labor income and capital income τw and τk , the optimization problem of this household reads as Vy,d (sy,d ) = max Vy,d (sy,d )Q(η, η ) ∀j ∈ {1, ...30 − 1} (6) u(cy,d ) + β cy,d ,ay,d. η ∈N e. where Vy,d (.) is the value function of a young household with deceased parents and sy,d is the vector of state variables in period j, which is given by sy,d = (ay,d , e, η j,e , j). (7). Agents maximize (6) subject to the budget constraint5 ay,d = (1 + r(1 − τk ))ay,d + (1 − τw )εej η j,e w − cy,d. (8). ay,d ≥ 0 The state space Sy,d of an household of type y, d thus includes four variables: own asset holdings, ay,d ∈ R+ , education level, e ∈ E, stochastic productivity, η j,e ∈ N j,e , and age j ∈ {1, ..., 30}. Notice that Sy,d = R+ × E × N e × {1, ..., 30}. Let P(E), P(N e ) and P{1, ..., 30} be the power sets of E, N e and {1, ..., 30}, respectively, and let B(R+ ) the Borel σ-algebra of R+ . It follows that Sy,d = B(R+ ) × P(E) × P(N e ) × P(J w ) is a σ-algebra on Sy,d and that My,d = (Sy,d , Sy,d ) is a measurable space. I will assume that the value function Vy,d :Sy,d → R and the policy functions cy,d :Sy,d → R+ and ay,d :Sy,d → R+ are measurable with respect to My,d . 5. Notice that for j = 30, the value function reads as ⎫ ⎧ ⎬ ⎨ Vy,d (sy,d ) = max Vp,1 (sp,1 )Q(η, η )Qinitial,e (η, di) u(cy,d ) + β ⎭ cy,d ,ay,d ⎩ e η ∈N. When j = 30, child households become parent household in j + 1. This implies that they observe their offspring ’s initial productivity level which becomes part of their state vector sp,1 .. 7.
(13) 3.1.2. Young households whose parents are alive. At any age j ∈ {1, ..., 30}, a household whose parents are still alive consume cy,a and have end-of-period wealth holdings of ay,a , where y, a denotes a young household whose parents are alive. Its parent household has wealth holdings of apy,a . Since the child does not know when the parent household dies, the value function is a weighted sum of the utility it receives if the parent household dies and the utility which is obtained if the parent continues to live for another period, where the parental survival probability ψj+30 serves as a weight. The optimization problem can thus be described by the following functional equation: . u(cy,a ) + β(1 − ψj+30 ) η ∈N e Vy,d (sy,d )Q(η, dη ) Vy,a (sy,a ) = max (9) +βψj+30 η ∈N e Vy,a (sy,a )Q(η, dη ) cy,a ,ay,a ∀j ∈ {1, ...30 − 1} 6 where ψj+30 is the survival probability of the parent household, Vy,a (sy,a ) denotes the value function given the state vector sy,a , where sy,a is described by j,e sy,a = (ay,a , ap y,a , e, η , j). (10). Notice that children observe only their parents end-of-period asset holdings. This implies that the law of motion of parental asset holdings is not part of the information set of the child household.7 The household maximizes (9) subject to its current period budget constraint ay,a = (1 + r(1 − τk ))ay,a + (1 − τw )εej η j,e w − cy,a. (11). ay,a ≥ 0 If the parent household dies in period j − 1, the flow budget constraint becomes ay,d = (1 + r(1 − τk ))(ay,a + apy,a ) + (1 − τw )εej η j,e w − cy,a. (12). ay,d ≥ 0 6. If j = 30, the child household knows that its parent household will die for sure in the current period. The Bellman equation thus reads as ⎫ ⎧ ⎬ ⎨ Vy,a (sy,a ) = max Vp,1 (sp,1 )Q(η, η )Qinitial,e (η, di) u(cy,d ) + β cy,a ,ay,a ⎩ ⎭ e η ∈N. 7. I also experimented with a model in which children use the policy function of their respective parents’ problem in order to update their information about expected bequests. This adds another to variables to the child household’s state space, namely the education and the productivity level of the parent households, thereby resulting in a dramatic increase in CPU time needed to solve the model. I found that parental asset holdings alone are sufficient to forecast future bequests. Including education and productivity did not change the child’s behavior at all.. 8.
(14) Because a child household keeps track of its parents wealth holding, I need to extend the state space Sy,a by apy,a ∈ R+ . The state space contains now two continuous variables, and is given by Sy,a = R+ × R+ × E × N e × {1, ..., 30}. Similar to the problem of a child household with deceased parents given above, I define a measurable space My,a = (Sy,a , Sy,a ), with respect to which Vy,a :Sy,a → R, cy,a :Sy,a → R+ and ay,a :Sy,a → R+ are measurable.. 3.2. Parent Households. Consider now a parent household, 31 ≤ j ≤ J max . A parent household works during the first 10 years and is retired afterwards. The household faces a declining survival probability, ψj < 1. In the following, I define the parent household’s problem in three different stages. 3.2.1. Parent Household, Working Vp,w (sp,w ) = max. cp,w ,ap,w. u(cp,w ) + βψj. . Vp,w (sp,w )Q(η, η ) ∀j ∈ {32, ...40}. (13). η ∈N e. where Vp,w (.) is the value function of a young household with deceased parents and sp,w is the vector of state variables in period j given by sp,w = (ap,w , e, η j,e , j). (14). Agents maximize (13) subject to the budget constraint ap,w = (1 + r(1 − τk ))ap,w + (1 − τw )εej η j,e w − cp,w. (15). ap,w ≥ 0 The state space is given by Sy,a = R+ × E × N e × {32, ...40}. I define a measurable space Mp,w = (Sp,w , Sp,w ), with respect to which Vp,w :Sp,w → R, cp,w :Sp,w → R+ and ap,w :Sp,w → R+ are measurable. 3.2.2. Parent Household, Retired. This household receives social security benefits, pen, and chooses consumption cp,r and its end-of-period wealth level ap,r . The optimization problem of this household can be written in recursive formulation as follows: Vp,r (sp,r ) = max u(cp,r ) + βψj Vp,r (sp,r ) (16) cp,r ,ap,r. ∀j ∈ {41, ...J max − 1} where Vp,r (sp,r ) is the value function, given the state vector sp,r . It follows that sp,r = (ap,r , j) 9. (17).
(15) The household maximizes (16) subject to ap,r = (1 + r(1 − τk ))ap,r + pen − cp,r. (18). ap,r ≥ 0 In the terminal period J max , (16) reduces to Vp,r (sp,r ) = max {u(cp,r )}. (19). cp,r ≤ (1 + r(1 − τk ))ap,r + pen. (20). cp,r. subject to The state space is now given by Sp,r = R+ × {41, ..., J max }. I construct a measurable space Mp,r = (Sp,r , Sp,r ), with respect to which I define Vp,r :Sp,r → R, cp,r :Sp,r → R+ and ap,r :Sp,r → R+ to be measurable. 3.2.3. Parent Household, First Period. In their first period (j = 31), parents incorporate the discounted lifetime utility of their children. They choose their own savings ap,1 and the transfers to their child household in such a way that their total utility is maximized. Transfers can be in form of assets (tra) and investment in education (ed). Recall that the education level is a binary variable, that is, ed ∈ {0, 1}, where ed = 0 if parents choose not to send their children to college and ed = 1 if parents send their children to college. Expressed in terms of a Bellman equation, the decision problem of a parent household at j = 31 reads as. u(cp ) + βψ31 Vp (sp ) Vp,1 (sp,1 ) = (21) max +ς (E [Vy,a (sy,a )|ed = 1] + E [Vy,a (sy,a )|ed = 0]) cp,1 ,ap,1 ,tra,ed where ς is the intergenerational discount factor. I allow for imperfect altruism, that is, 0 ≤ ς ≤ 1. If ς = 0, parents care only about their own utility. The model thus nests a pure life cycle economy (ς = 0) and a dynastic model (ς = 1) as extreme cases. Both Laitner (2001) and Nishiyama (2002) show that the observable flow of transfers is consistent with an intermediate case. Clearly, the degree of altruism matters for parental transfer behavior. Notice that it influences only the total amount of resources which is transferred, but does not have any effect on the division into education investment and financial transfers. Vp,1 (sp,1 ) is the value function for a given state vector sp,1 , where sp,1 = (ap,1 , e, η j,e , i). (22). Notice that the initial productivity level i becomes part of the parent household’s state space because the child may drop out before graduating. i determines the probability to drop out. Together with the fact that the income stream is stochastic, this implies that investment in college risky. Therefore, whether parents invest in the children’s education beyond high school depends on the difference between E [Vy,a (sy,a )|ed = 1], the expected utility from 10.
(16) investing, and E [Vy,a (sy,a )|ed = 0], the value if they do not invest. More precisely, the expected utility from investing is given by. λ(i)Vy,a (tra, ap,1 , col, η 1,col , 1) E [Vy,a (sy,a )|ed = 1] = (23) +(1 − λ(i))Vy,a (tra, ap,1 , hs, η 1,hs , 1) where λ(i) is the probability that the child household completes college education successfully. The expected utility is thus a weighted average of the expected lifetime utility if the child completes education and of the expected value of the household if it does not complete education. If the child household enters college but does not complete college education, the child household faces the same value function as a child household which does not enter college education. The parental budget constraint is given by ap,1 = (1 + r(1 − τk ))ap,1 + (1 − τw )εej η j,e w + ν|ed=1 (ap,1 , εej η j,e w) − tra − κ|ed=1 − cp,1 (24) ap,1 ≥ 0 where ν denotes the college subsidy the household receives if ed = 1, κ denotes the fixed college expenses that the parent household pays. The subsidy level ν is a function of family resources available for college investment. In the U.S., most programs are targeted towards students of low income families. In this case, the amount of subsidies depends negatively on the amount of family resources (see e.g. Feldstein (1995)). Note that children may also receive end-of-life bequests. Because average bequests are higher the more the parent saves in period j = 31, the value function of the child,Vy,a (sy,a ), is increasing and concave in ap,1 . Because the parent household incorporates Vy,a (sy,a ) in its decision problem in j = 31, it also incorporates the utility from leaving bequests. This establishes a trade-off between transferring resources in the form of inter vivos transfers or in the form of end-of-life bequests. Note that this mechanism would also work in the presence of a perfect annuity market. Using the fact that the state space of parents in their first period is given by Sp,1 = R+ × E × N e × {1, 2, ..., i}, I construct a σ-algebra on Sp,1 as Sp,1 = B(R+ )×P({1, 2, ..., i}) ×P(E) × P(N e ) where P({1, 2, ..., i}) is the power set of {1, 2, ..., i}. Mp,1 = (Sp,1 , Sp,1 ) is then a measurable space, which implies that Vp,1 :Sp,1 → R, cp,1 :Sp,1 → R+ , ap,1 :Sp → R+ , tra :Sp,1 → R+ and ed :Sp,1 → {0, 1} are measurable on Mp,1 .. 3.3. The Firm’s Problem. There is a continuum of firms, which I normalize to have total measure one. Firms are competitive and take all prices as given. Thus, I assume a single representative firm. This representative firm uses aggregate physical capital K and aggregate labor measured in efficiency units L to produce a single identical output good Y . The profit-maximizing conditions of the representative firm are r + δ = FK (K, L) (25) w = FL (K, L) where F (K, L) is a constant returns to scale production function. 11. (26).
(17) 3.4. The Government’s Problem. The infinitely lived government administers the pension system and distributes college subsidies. The government finances pension benefits and subsidies by issuing a payroll tax on labor and capital income. I impose that the budget of the government has to be balanced in each period. Let Φ be a probability measure defined over the measurable spaces My,d , My,a , Mp,1 ,Mp,w and Mp,r , which result from the household problem as stated above.8 The government computes old-age pension benefits, pen, as the average lifetime income of a high-school graduate times a social security replacement ratio: j,hs hs j,hs w R+ ×{hs}×N hs ×J w εhs η dΦ + ε η dΦ j R ×{hs}×N hs ×J w j + pen = rep (27) dΦ + R+ ×{hs}×N hs ×J w dΦ R+ ×{hs}×N hs ×J w I assume that tax rate levied on capital, τk , is determined exogenously. The government’s problem thus reduces to adjusting tax rate on labor income τw such that budget is balanced: pen S dΦ + Ξ − τk rK p,r (28) τw = wL where the total amount of college subsidies Ξ is given by Ξ= ν|ed=1 dΦ. (29). Sp,1. 4. Definition of a Stationary Competitive Equilibrium. I now define the equilibrium that I study: Definition 1 Given a replacement rate, rep, and a tax rate for capital income, τk , a college subsidy rule ν, a Stationary Recursive Competitive Equilibrium is a set of functions Vy,d (sy,d ), Vy,a (sy,a ), Vp,1 (sp,1 ), Vp,w (sp,w ), Vp (sp ), cy,d (sy,d ), cy,a (sy,a ), cp,1 (sp,1 ), cp,w (sp,w ), cp (sp ), ay,d (sy,d ), ay,a (sy,a ), ap,1 (sp,1 ), ap,w (sp,w ), ap (sp ), tra(sp,1 ), ed(sp,1 ), non-negative prices of physical capital and of effective labor, {r, w}, and set of probability measures on the state spaces of the respective household problem as defined in sections (3.1.2)-(3.2.3) such that the following hold: 1. Given prices and policies, Vy,d (sy,d ), Vy,a (sy,a ), Vp,1 (sp,1 ), Vp,w (sp,w ) and Vp (sp ) are the solution to the household problem outlined in (3.1.2)-(3.2.3) with cy,d (sy,d ), cy,a (sy,a ), cp,1 (sp,1 ), cp,w (sp,w ), cp (sp ), ay,d (sy,d ), ay,a (sy,a ), ap,1 (sp,1 ), ap,w (sp,w ), ap (sp ), tra(sp,1 ), ed(sp,1 ) being the associated policy functions. 8 Notice that the total population size is normalized to one. The probability measure thus defines the number of people (or equivalently, the total population share) facing a specific endowment with state variables.. 12.
(18) 2. The prices r and w solve the firm’s problem (25) and (26). 3. The government policies satisfy (27), (28) and (29). 4. Markets for physical capital, labor in efficiency units and the consumption good clear: a (s )dΦ + a (s )dΦ y,d y,a Sy,d y,d Sy,a y,a (30) K= + Sp,1 ap,1 (sp,1 )dΦ + Sp,w ap,w (sp,w )dΦ + Sp ap (sp )dΦ. . where C=. e j,e e j,e ε η dΦ + ε η dΦ j j S S y,d y,a L= + Sp,1 εej η j,e dΦ + Sp,w εej η j,e dΦ. (31). C + [K − (1 − δ)K] + T + I − Ξ = F (K, L). (32). c (s )dΦ + c (s )dΦ y,d y,d y,a y,a Sy,a Sy,d + Sp,1 cp,1 (sp,1 )dΦ + Sp,w cp,w (sp,w )dΦ + Sp cp (sp )dΦ T = tra(sp,1 )dΦ . Sp,1. I = κ(1 − ν). (33) (34). Sp,1. ed(sp,1 )dΦ. (35). 5. The Aggregate Law of Motion is stationary: Φ = H(Φ). (36). The function H is generated by the policy functions ay,d (sy,d ), ay,a (sy,a ), ap,1 (sp,1 ), ap (sp ), tra(sp,1 ), ed(sp,1 ), the Markov process Q(η e , N e ) and the transmission matrix Qinitial,e (i, i ∈ I = {1, 2, ..., n}) and can be written explicitly as (a) For all sets (A, Ap , E, N e , J ) with J = {2, ..., 30} such that (A, Ap , E, N , J ) ∈ S y,a , the measure of agents whose parents are alive is given by Φa = Py,a (sy,a , (A, Ap , E, N e , J ))dΦa (37) Sy,a. where Py,a (sy,a , (A, Ap , E, N e , J )) ψ(j+30) Q(η, η ) if ay,a (sy,a ) ∈ A, ap (sp ) ∈ Ap , e = e ∈ E, j + 1 ∈ J ∈N e η = 0 otherwise Py,a (sy,a , (A, Ap , E, N e , J )) is the transition function. It gives the probability that an agent with endowment sy,a at age j ends up in j+1 with asset holdings ay,a ∈ A, productivity state η ∈ N e and parental asset holdings ap ∈ Ap . The education level remains constant. 13.
(19) (b) For all sets (A, E, N e , J ) with J = {2, ..., 30} such that (A, E, N , J ) ∈ S y,d , the measure of agents with deceased parents is given by e P (s , (A, E, N , J ))dΦ + d Sy,d y,d y,d Φd = (38) p e (1 − ψ ) P (s , (A, A , E, N , J ))dΦa j+30 y,a y,a Sy,a where Py,d (sy,d , (A, E, N e , J )) Q(η, η ) if ay,a (sy,a ) ∈ A, e = e ∈ E, j + 1 ∈ J ∈N e η = 0 otherwise As fraction (1 − ψj+30 ) of parents dies in period j, Φd incorporates the measure of young agents whose parents died in the previous period. (c) For all sets (A, I, J ) with J = {31} such that (A, I, J ) ∈ S p,1 , the measure of parent households in j = 31 is given by P (s , (A, I, J ))dΦ p,1 y,d d Sy,d Φp,1 = (39) + Sy,a Pp,1 (sy,a , (A, I, J ))dΦa where Pp,1 (sy,d , (A, I, J )) initial,e Q (i, i ) if ay,a (sy,a ) ∈ A, j + 1 ∈ J = i ∈I 0 otherwise and Pp,1 (sy,d , (A, I, J )) follows straightforwardly. Pp,1 (., (A, I, J )) shows the transition from child households to parent households. The measure of parent households collects all child households. (d) The measure of parent households while working is generated in a similar fashion. (e) For all sets (A, Ap , E, N e , J ) with J = {1} such that (A, Ap , E, N , J ) ∈ S y,a , the measure of agents in their first period is given by initial initial Φ = Pp,1 (sp,1 , (A, Ap , E, N e , J ))dΦp,1 (40) Sp,1. initial (sp,1 , (A, Ap , E, N e , J )) is given by ap,1 (sp,1 ), tra(sp,1 ) and ed(sp,1 ) where Pp,1 where ap,1 (sp,1 ) ∈ A, tra(sp,1 ) ∈ Ap and ed(sp,1 ) ∈ E.. (f ) The measure of agents during retirement is generated by the policy function ap (sp ) where ap (sp ) ∈ A. A few remarks regarding the equilibrium conditions are in order. (30) and (31) state that aggregate physical capital and labor measured in efficiency units follow from aggregating the respective holdings of each agent and weighting them appropriately. (32) requires that the good market clears, i.e. that the demand for goods, which is shown on the left-hand side, 14.
(20) is equal to the supply of goods. The term [K − (1 − δ)K] on the left-hand side determines the amount of investment that is necessary to keep the aggregate capital stock constant, whereas I and T are aggregate college expenditures and transfers in stationary state, respectively. (36) requires stationarity of the probability measure Φ. The function H is the transition function which determines the probability that an agent will end up with a certain combination of state variables tomorrow, given his endowment with state variables today. Notice that the stationarity condition requires that child households are (on average) ’identical’ to their parents in the sense that they reproduce their parent household’s distribution once they become parents themselves. This in turn implies that the distribution of transfers and inheritances that child households receive is consistent with the distribution of transfers that is actually left by parent households. I present more details about the computational procedure in the appendix.. 5. Parametrization and Calibration. I calibrate parameter values of the benchmark economy to represent relevant features of the U.S. economy as closely as possible. It will be assumed that the length of one unit of time in the model economy corresponds to a calendar year. The targets that I choose for the benchmark economy describe the U.S. economy around 1980. I therefore label this benchmark case ’economy 1980’. In order to compare the change of enrolment patterns over time, I define a second steady-state which I denote as ’economy 2000’.. 5.1 5.1.1. Economy 1980 Technology, Demographics and Preferences 1−γ. I assume that the utility from consumption in each period is given by u(c) = c1−γ . Production is assumed to follow the aggregate production function F (K, L) = K α L1−α . I set the capital share in income (α) equal to 0.36, as estimated by Prescott (1986). Following Imrohoroglu et. al. (1995) and Heer (2001), I assume that capital depreciates at an annual rate of 8 percent. The conditional survival probability ψj is taken from the National Vital Statistics Report, Vol. 53, No. 6 (2004) and refers to the conditional survival probability for the U.S. population. Only values between age 53 and age 82 are used. I assume that the survival probability is zero for agents at the age of 83. The survival probability for households that are younger than 53 years is assumed to be equal to 1.9 The preference parameter γ determines the relative risk aversion and is the inverse relation to the intertemporal elasticity of substitution. I follow Attanasio (1999) and Gourinchas and Parker (2002) who estimate γ using consumption data and find a value of 1.5. This value is well in the interval of 1 to 3 commonly used in the literature. The two main parameters that govern the accumulation of wealth and transfer behavior the discount factor β and the intergenerational discount factor ς - are calibrated jointly such 9. The actual survival probability before 53 is close to 1. See the National Vital Statistics Report.. 15.
(21) that the baseline economy is consistent with the wealth-income ratio and the relative size of intergenerational transfers in the U.S. economy in 1980. Gale and Scholz (1994) compute a ratio of inter vivos transfers to total wealth of 0.28 percent from the 1983 and 1986 Survey of Consumer Finances. This number comprises financial non-college support to children. The resulting ς is 0.7, which implies that for the benchmark economy to be consistent with the transfer flows observable in the U.S. economy, a parent household would have to consider the utility of a child household 30 percent less than it considers its own utility. This is in line with results obtained from Nishiyama (2002) who uses an altruistic framework to explain the observable degree of wealth inequality in U.S. economy. 5.1.2. Earnings Process. I assume that the process that governs the productivity shocks η j,e follow an AR(1) process with persistence parameter ρhs for high school graduates and ρcol for college graduates. The variance of the innovations are σ hs and σ col , respectively. These parameters are estimated by Hubbard et al. (1995)(HSZ in the following) from the 1982 to 1986 Panel Study of Income Dynamics (PSID). They find that high school graduates have a lower earnings persistence and a higher variance (ρhs = 0.946, σ hs = 0.025) compared to college graduates (ρcol = 0.955, σ col = 0.016). It should be noted that both estimates are rather conservative as HSZ use the combined labor income of the husband and wife (if married) plus unemployment insurance for their estimates. When I approximate the earnings process with a four-state Markov process using the procedure proposed by Tauchen and Hussey (1991), I find that the transition matrices for high school and college graduates are nearly indistinguishable. I also take the average age-efficiency profile εej from HSZ, which gives us an estimate of the college premium for different age groups. The authors find that earnings are more peaked for college families, which is in line with findings from other empirical studies. Different from the model estimated by HSZ, we endogenize the college enrolment decision. This implies that in equilibrium, college graduates are more likely to have positive deviations with the respect to the average age profile, because more productive children (measured in terms of their first draw from the productivity distribution) are more likely to attend college. This is not reflected in the estimation of the mean age-earnings profile of HSZ. I thus adjust the age profile for college graduates in the model downwards, such that the average college premium after selection coincides in both models. For the economy 1980, I also adjust their estimates for the earnings variance. The reason is that HSZ estimate their model for the beginning of the 1980’s. Parents who decide upon transfers in the beginning of the 1980’s accumulated their wealth in the 1970’s or even earlier. Gottschalk and Moffitt (1994) find that the variance of both permanent and transitory earnings increased by 40 percent between the two decades. For the economy 1980, I thus use a σ hs of 0.015 and σ col of 0.01.. 16.
(22) 5.1.3. College Completion and Cost of College. I assume that the probability of college completion λ(i) is an increasing function of the initial productivity state i. In particular, I assume λ(i) = d + a(i − 1). (41). d, a ≥ 0 Recall that I approximate the AR(1) process with a 4-state Markov chain; I therefore have a grid with 4 points that represent the different productivity levels. Consequently, the parameter d governs the completion probability for child households with low ability (i = 1). d thus governs the expected return associated with college investment. I set d such that the college participation rate of low ability students with parents in the highest income quartile is 0.3. This is also the enrolment share of low-ability children from families in the highest income quartile in the NLSY79 as reported by Belley and Lochner (2007).10 I use families from the highest income quartile as a calibration target because financial constraints are not very likely to have an impact on their college enrolment decision (Carneiro and Heckman (2002)). Instead, I impose that their decision is solely based on the expected return, which is governed by d. For d = 0.32, the benchmark steady-state replicates the enrolment share of high-income families with children of low ability. This implies that these students graduate with a probability of 32 percent. Two additional parameters influence the college investment behavior, the tuition costs κ and the slope parameter a. I calibrate these parameters jointly such that the model is consistent with an overall dropout probability of 50 percent (Restuccia and Urrutia (2004)) and a fraction of college graduates of 25 percent. I obtain a κ of 0.95 and an a of 0.07. In line with U.S. evidence, the model implies that total college expenses are approximately equal to per-capita GDP (see e.g. Collegeboard (2005) or Gallipoli et al. (2006)). A slope parameter a of 0.07 implies that high-ability children have a 21 percent higher change of graduating from college than low-ability children. 5.1.4. Transmission of Initial Productivity. In the data, there is a high degree of persistence in economic outcomes across generations. Inheritability of genetic traits, the family environment and early education all matter for explaining different levels of pre-college ability levels Restuccia and Urrutia (2004). Following Keane and Wolpin (2001), I assume that the transmission of initial productivity levels depends solely on the level of parental education. Technically, I generate a positive link by assuming that parents transmit part of their productivity shock at age j = 30 to their children, who enter the economy when parents turn to j = 31. 10. Belley and Lochner (2007) use the Armed Forces Qualification Test (AFQT) as a proxy for ability. AFQT test scores are a widely used measure of cognitive achievement by social scientists using the NLSY and are strongly correlated with positive outcomes like education and post-school earnings. See their footnote 2 for further references. 17.
(23) Because transmission depends on parental education, I define two separate transition matrices for parents with high school and college education, Qinitial,hs and Qinitial,col . Let col pcol ip ,ic ∀{1, ..., 4} be an element of the transition matrix of college graduates. Then, pip ,ic is the probability that a college educated parent household of age j = 30 is of productivity ip , while the child receives an initial productivity of ic . In order to achieve a positive link across hs c col hs generations, it needs to be that pcol ip ,ic ≥ pip ,ic for ’high’ levels of i and pip ,ic ≤ pip ,ic for ’low’ c levels i . In addition, I require that pip ,ic ≥ 0 to ensure that there are non-trivial percentages in all productivity levels. Moreover, the probabilities in each row of the transition matrix have to sum to 1, ic pip ,ic = 1. Limited by these conditions, I model both transition matrices as linear combinations between an identity matrix and a matrix which rows consist of an additive sequence. For college graduates, this sequence has starting value 0, increment (n−1)n and n elements, where 2 n is the number of productivity shocks. Let π be the weight of this matrix and (1 − π) the weight of the identity matrix. Then, pip ,ic ≥ 0 requires π ∈ (0, 1). π is calibrated such that the model reproduces the correlation of college education across generations. Data from NLSY 79 reported by Keane and Wolpin (2001), Table 4, suggests that this correlation is between 0.28 and 0.38, depending on the youth’s level of completed schooling at age 16. The correlation of education college attainment is significantly weaker if I consider only parentchild pairs for which the children’s schooling level at the age of 16 is similar. Since I do not model differences pre-tertiary education, I choose π such that the model implies a correlation of 0.31. 5.1.5. College Subsidies and Taxes. Parents who send their children to college receive a government subsidy ν|ed=1 (ap,1 , εej η j,e w) for each unit of expenditure in college education. The subsidy is a function of current income and asset holdings. In the U.S., the calculation of the subsidy is based on an estimate of the student’s family ability to pay the cost of college. This estimate is based on estimates of ’discretionary income’ and ’available assets’ Feldstein (1995). I approximate discretionary income as the sum of labor and capital income, net of taxes. Available assets are calculated as the difference between current wealth holdings and a wealth level that is deemed to maintain the current standard of living, which I approximate by the average asset holdings in the economy, called a. These two measures are then combined by adding 12 percent of the available net assets to the discretionary income, see Feldstein (1995). The key point of the exercise is that every extra dollar of savings raises the amount of available resources, which decreases the subsidy. Feldstein (1995) points out that this indirect savings tax may generate strong disincentives for the accumulation of wealth. For simplicity and because this specification is common in the literature, I assume that the subsidy level is linearly decreasing in the level of parental resources: ν = max(ν0 − ν1 (max(0, ap,1 − a) + (1 − τw ) rap,1 (1 − τw ) εej η j,e w), 0) ν0 , ν1 ≥ 0 18. (42).
(24) I calibrate ν0 and ν1 such that (i) the ratio of college subsidies to total college expenses, the subsidy rate, is 0.4, as reported by the OECD (see Akyol and Athreya (2005), Figure 1) and (ii) the subsidy does not cover more than 50 percent of κ, the total college expenses an individual household has to pay, see Keane and Wolpin (2001). This implies estimates of ν0 of 0.57 and of ν1 of 0.1. Following De Nardi (2004), I use a capital income tax rate τK of 0.2 and a replacement rate for pension benefits of 0.4. Finally, I adjust the tax rate on labor income τw such that the government budget (28) is balanced. This results in a tax rate of 15 percent. The results are summarized in Table 2.. 5.2. Economy 2000. I adjust the average college premium, the earnings process and the tuition fees in order to account for the increase in between-group inequality, within-group inequality and the doubling of the college expenses (in real terms, see Collegeboard (2005)). All other parameter values are left unchanged. The college premium increases by 30 percentage points (from 40 percent to 70 percent compared to our benchmark case (see Katz and Autor (1999)).. 6. Results. In this section, I analyze the quantitative behavior of our benchmark economy. In particular, I use my model as a measurement tool in order to evaluate to what extend borrowing constraints are binding.. 6.1. Economy 1980: How Important are Borrowing Constraints?. In this section, I show that the fraction of households that is borrowing constrained in their college decision is 18 percent, which is substantially higher than what estimates from the empirical literature suggest. Carneiro and Heckman (2002) find that at most 8 percent of the population are borrowing constrained in the short-run sense. I argue that the difference is due to the measurement of long-term factors and the fact that parents are imperfectly altruistic. If I apply their methodology to the data generated by the model, my results are broadly consistent with their findings. 6.1.1. The Fraction of Constrained Households. In order to measure the fraction of constrained young households, I start with an experiment in which I allow parents to borrow up the total college expenses. I implement the loan as a transfer from the government to all parents before they decide on how much to invest in their children. In order to keep its budget balanced, the government in turn collects the resources from the child households. The debt contract takes the form of a redeemable loan, for which the annual redemption sum is fixed and independent of the child household’s income. Now, I ’force’ parents to use the loan for their children, either in form of financial transfers or in form of college investment or both. Parents cannot use the additional resources for their 19.
(25) own consumption. It is important to notice that this experiment is equivalent to a scenario where the child households are offered a loan directly, and where they decide themselves whether they want to invest in human capital or in financial assets.11 If the total loan amount is transferred in form of financial assets, the net present value of the loan is zero by construction.12 I find that the second experiment raises the total college enrolment rate to 75 percent, an increase of 18 percentage points relative to the benchmark economy. From this it follows that the presence of borrowing constraints for college education is associated with a decrease in the college enrolment rates of 18 percent relative to an economy where enrolment is dictated solely by the expected value of going to college. This result exceeds the findings from the empirical literature considerably. Carneiro and Heckman (2002) conclude that the fraction of constrained households in the population is at most 8 percent. In the next two sections, I shed further light on the difference between my results and theirs. I show that once I apply their methodology to the data generated by my model, the results broadly coincide. 6.1.2. Enrolment Gap. I now examine how the model compares to the data as regards to enrolment gaps between income groups. For the 1980 economy, I compute the college enrolment rate by family income and ability level. Figure 3 plots the results. The empirical counterpart is taken from Belley and Lochner (2007), Figure 2a, who study data from the NLSY79. Figure 4 shows their results. I find that - both in the model and in the data - college enrolment increases with ability level. In the model, this is an immediate consequence of the assumption that more able children have a higher likelihood to graduate from college, which makes college investment more profitable for their parents. These plots also indicate a subsidiary, but quantitatively important role for family income in accounting for college entry, which one might think of as indicating the importance of borrowing constraints. In an influential paper, Carneiro and Heckman (2002) claim that enrolment gaps with respect to family income are not very informative about the strength of borrowing constraints. They argue that one needs to distinguish between short-term borrowing constraints, which are created by short-term cash-flow problems when a child is on the threshold of college enrolment, and long-term borrowing constraints related to a family’s ability to finance education through a youth’s childhood. Only the short-term constraints are of relevance for public policy in my framework, as they can be addressed directly with policy measures such as a different college subsidy scheme. Family income, which is measured when the child is on the threshold of college entry, thus captures shortterm as well as long-term constraints. Carneiro and Heckman control for long-term factors by including parental education and a set of other family related variables in addition to a measure of academic ability when 11 Because parents choose the optimal mix between financial transfers and college investment such that they maximize their own utility and their children’s utility is part of their total utility, the parental decision about the optimal division of the loan coincides with the decision the offspring would take. 12 I expect that low-ability children receive a greater share of the loan in terms of financial transfers.. 20.
(26) computing the enrolment gaps. They find that gaps in college entry by family income narrow significantly after controlling for long-run borrowing constraints (see Figure 5 in their paper). In Table 5, I report the enrolment gaps with respect to the highest income quartile generated by the baseline economy. Each column represents a different ability quartile. Panel A of Table 5 documents the enrolment gaps corresponding to Figure 3. Panel B and C give the enrolment gaps after controlling for the parental education level (high school or college, respectively). In line with Carneiro and Heckman (2002), I find that enrolment gaps narrow after controlling for long-term factors, in my case ability and parental schooling. Table 5 reveals that enrolment gaps for children from college graduated parents differ very little across different income groups. Carneiro and Heckman (2002) interpret these enrolment gaps as the fraction of the population that cannot attend college because of financial constraints. They conclude that at most 8 percent of the population is constrained in their college decision. I repeat their analysis and weight the enrolment gaps documented in Table 5 with the fraction of the total population in the respective ability and income quartile. I find that the maximum fraction constrained is 14 percent if I condition on high school education (Panel B) and 11 percent if I control for college education (Panel C). Without controlling for education, the estimated share would have been considerably higher (20 percent, panel A) which confirms that incorporating long-term factors decreases the conjectured role of borrowing constraints, as argued by Carneiro and Heckman (2002). Above I argued that removing the borrowing constraint for college enrolment leads to an 18 increase in the enrolment rate. Thus, the model-based estimate of the extent to which borrowing constraints adversely affect college entry is substantially higher than the econometric estimate derived from controlling for long-term factors. I will now show that this disparity is due to imperfect altruism on the part of parental households which implies that young and able households may receive insufficient transfers even if their parents could afford to send them to college. 6.1.3. The Role of Imperfect Altruism. In order to gauge the importance of imperfect altruism, I repeat the experiment from Section 6.1.1 but let parents decide how to spend the additional resources provided by the government. Thus, comparing this experiment to the ’forced’ experiment reported in Table 4, allows me to check how imperfect altruism affects the extent of borrowing constraints for prospective college entrants. I will refer to this experiment as ’free disposal’. The fact that parents are imperfectly altruistic implies that parents weight the disutility the child suffers from repaying the loan less than their utility gain they obtain by using part of the loan for their own consumption purposes. I thus expect that parents do not provide the total loan amount to their children.13 The ’free disposal’ experiment therefore mainly illustrates the 13. Because of imperfect capital markets, parents might also be borrowing constrained for their own consumption purposes. Since parents are already in an advanced stage of the life cycle when transfers take place, I do not expect constraints on parental consumption to be binding for a larger fraction of families.. 21.
(27) extend to which imperfect altruism biases our measured fraction of constrained families. Table 4 reports the total share of students that engage in college education. I find that the fraction of college students in each cohort increase from 57 percent to 62 percent when I allow for borrowing. Compared to the increase of 18 percent that one could observe in the ’forced’ experiment above, this share appears to be relatively small. In order to understand the differences, it is interesting to compare the Figures 3, 5 and 6. I find that in the experiment with ’forced’ credit (Figure 6), the enrolment rates increase not only for households from the lower end of the income distribution, but also for rich households. I do not observe this pattern in the ’free-disposal’ experiment (Figure 5), which suggest that enrolment rates rise because the ’forced’ experiment eliminates all effects of imperfect altruism. Hence, ignoring the possibility of imperfect altruism may bias the estimated fraction of constrained households downwards. The results show that, even though the average enrolment gap measured in the model is in line with empirical results by Carneiro and Heckman (2002), the true fraction of constrained households is much larger as even children from high-income family may not receive the sufficient amount of transfers needed to go to college. 6.1.4. The Role of Parental Education as a Long-Run Factor. Conditioning on parental education has a significant impact on observable enrolment patterns, implying that there is a strong link between parental education and children’s college attendance. Apart from the fact that college graduated parents are richer, they also have, by assumption, smarter children. However, Table 6 documents that even after controlling for ability and family income quartile, offspring from college educated families have a 4.7 percent higher change of being enrolled in college than descendants from high school educated families (Table 6, first row). This difference stems from the fact that education not only determines the level of family income but also the level of parental wealth. In the empirical literature, family income is usually solely observed in a specific year. Wealth in turn is determined by permanent income, which may only be weakly related to the level of income around college age. The second row of Table 6 accounts for this effect. Here, I compute the average difference between the enrolment rates of college graduates and high school graduates, controlling for the quartile of family income, ability and assets. The results of the first and the second row are extremely similar, suggesting that differences in asset holdings - after controlling for family income - do not explain the gap in college enrolment between children of differently educated parents. The findings contribute to the debate in the literature on whether parental education should be used as a measure for long-term borrowing constraints (Carneiro and Heckman (2002), Kane (2006)). To the extent that parents can help financing their children’s education with current income or accumulated assets, conditioning on parental education may lead to an understatement of the role of short-term constraints (Kane 2006, p. 1394). The results suggest that this is not the case; parental education seems to have an influence on college going, which is independent of the wealth effect. 22.
(28) Neither differences in parental endowment nor differences in children’s ability explain fully why high school educated parents provide less support for their offspring’s college education. Instead, the differences stem from different expectations about the future. High school graduates are exposed to a higher earnings risk and lower average earnings, even after the time their children left home. Indeed, I find that high school graduates have higher savings than college graduates at age 53, the age at which parent households decide about transfers. In Tables 7 and 8, I compute average savings for different ability, income and wealth quartiles, differentiated by the education level of the household. The results convey a clear message: high school graduates at age 53 tend to save more than college graduates at this age. Thus, in conclusion, I find that large fraction of borrowing constrained households measured in my model compared to the literature can be explained by (i) the fact that parents are imperfectly altruistic, which implies that even children from high-income families may not receive enough parental funding and (ii) the high school educated parents save more and transfer less than their college educated counterparts, even if their children are equally well prepared for college.. 6.2 6.2.1. Economy 2000: Have borrowing constraints become more limiting? Have Borrowing Constraints Become more Limiting?. In order to analyze to what extent borrowing constraints have become more binding as the economic environment changed between 1980 and 2000, I repeat the ’free-disposal’ as well as the ’forced’ experiment for the 2000 economy. The ’free-disposal’ experiment for the economy 2000 indicates that the share of college students increases from 60 percent to 85 percent, which is a change of 25 percentage points (see Table 4). This result is in stark contrast to the result for the economy 1980, for which enrolment increased by only 5 percentage points. Comparison of the college enrolment rates for the tow different economies, see Figures 5 and 9 reveals that the low-ability students make the difference. While these agents’ college enrolment decisions are approximately unaffected by the provision of loans in the 1980 economy, this policy increases their enrolment rates significantly in the 2000 economy. This indicates that – due to the increase in the college premium – college education becomes more profitable for this group of students. As indicated by the enrolment patterns in the previous section, only rich parents are willing to take advantage of this and invest in their low-ability children. Despite the surge in the college premium, parents with lower income, instead, do not find it advantageous to transfer sufficient funds to their offspring. Applying the ’forced’ credit experiment to the economy 2000 reveals the full extent to which lack of parental transfers (and thus borrowing constraints) limit college enrolment. In this alternative experiment, enrolment goes up to approximately 100 percent. That is, around 40 percent of the population are constrained in their college decision, compared to. 23.
(29) 18 percent in the economy 1980.14 Thus, the recent changes in the economic environment in the U.S. generate a larger fraction of constrained households. In order to understand why, I present the changes in the enrolment patterns between 1980 and 2000 in the next section. 6.2.2. Enrolment Rates. I now analyze to what extend the increase in the college premium, the tuition fees and the variance of the productivity process have affected the college enrolment for different ability and family income quartiles. Figure 7 shows the enrolment rates obtained from our model economy 2000, while the empirical counterpart from the NLSY97 is displayed in Figure 8 (see Belley and Lochner (2007), Figure 2b). In line with their observations, I find that the role of ability did not change with respect to the economy 1980. Also consistent with the data, there are two striking differences between the economies of 1980 and 2000: 1. Enrolment rates are higher for the economy 2000. This suggests that in the aggregate, the rise in the rate of return on tertiary education more than outweighs the increase in risk and the higher price of tuition. 2. Enrolment gaps between different family income groups have widened over time, in particular for the low-ability students. This holds even after controlling for parental education (see Table 10). To better understand the increase in the enrolment gap for low-ability students, I compute the share of college students from the lowest ability for the economy 1980 and the economy 2000. I find that this fraction has more than doubled from 1.8 percent to 5 percent (see Table 4). This increase could indicate that the number of high income families with low ability children rose since high income families are more prone to send their offspring to college. However, our results reveal that the number of families from the top income quartile that have children with low ability actually declined from 2.4 to 1.3 percent. Consequently, the rise of the fraction of low-ability college students must be due to the increase in the college premium which made college investment more attractive, even for low-ability students. Next, I compute the average savings for different income, wealth and ability quartiles, using the policy functions and the steady-state distribution of agents generated by our economy 2000 for agents at age 53. The results are shown in Tables 10 and 11. If I compare the difference between high school and college graduates for 1980 (tables 7 and 8) with the differences in 2000, I find that high school graduated parents now save even more compared to their college graduated counterparts, all other things equal. The increase appears to be more pronounced for parents with children in the lowest ability quartile. 14. It is also interesting to note that the model implies that the share of students who drop out from college without a degree increased, albeit only slightly, when one compares the baseline economy to the economy 2000. This rise is an immediate consequence of the fact that the share of low-ability students increased. The findings thus provide an explanation for the changing dynamic between college enrolment and college completion, which is documented by Turner (2004). See Table 4. 24.
(30) Strikingly, savings of high school graduates relative to college graduates increases, after controlling income and wealth. This is due to the increase in the variance of earnings shocks, which I use in order to simulate the increment in the within-group inequality. This rise in uncertainty has a stronger impact on high school graduates, as labor earnings comprise a bigger share of total income for that group. Therefore, the need for precautionary savings is higher for the high school group, which causes them to keep more resources to secure their own future. This result is reinforced by the fact that high school graduated parents are more likely to have low-ability children, which reduces the expected return from investing. In addition, the rise in tuition fees has made college expenses even more expensive, reducing the incentives for the poor to invest in their children. In conclusion, I find that the recent changes in the economic environment generate a stronger link between family income and college enrolment in the model, which is in line with the data. The model predict that financial constraint have become more limiting over the course of time. In the model, this stems from the fact that the rise in the college premium implies that more young household are willing to go to college. Only the rich parents, however, can take advantage of this and invest. The others are hampered by the increase in within-group inequality, which requires more savings for their own future, and the increment of the tuition fees.. 6.3. Testable Implications. A key insight from the analysis above is that, according to my model, parents at age 53 with high school education save more and transfer less to their offspring than college graduates at the same age. I will now examine to empirical relevance of this aspect of my analysis. I address this issue by computing education specific savings and transfers from the 1986 Survey of Consumer Finances (SCF). 6.3.1. The Trade-Off between Transfers and Savings in the Data. The SCF is a household survey conducted on a triennial basis. It consists of a cross-section of U.S. households, with the exception of the waves in 1983 and 1986 which contain repeated cross-sections. This allows me to observe household savings between 1983 and 1986. Moreover, the 1986 wave also ask extensively about household’s transfer behavior, and has therefore become a standard reference with respect to parental inter-vivos transfers (see e.g. Gale and Scholz (1994)). I compute total transfers given by a household as the sum of all monetary transfers and college expenses, which are reported separately in the SCF.15 I use savings in constant prices accumulated between 1983 and 1986. In order to be consistent with the model, I only consider those households that are between 45 and 65 years old, and that have at least one child. As Table 12 shows, college graduates have on average higher savings and transfer more resources (column 1), as one would expect from the fact that college graduates are on average more affluent and have higher income than high school 15. The 1986 SCF only reports transfers if the transfer amount is above 3000 US-Dollar.. 25.
Figura
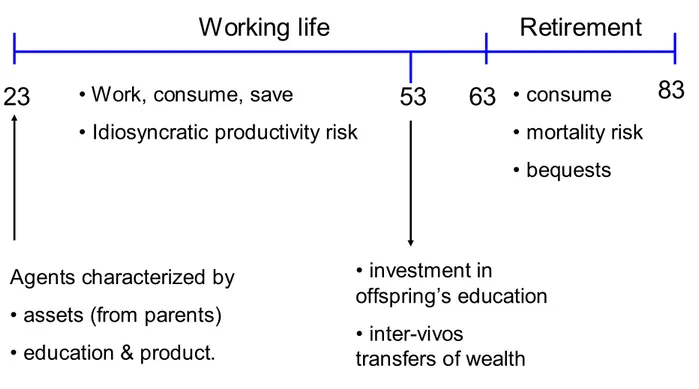


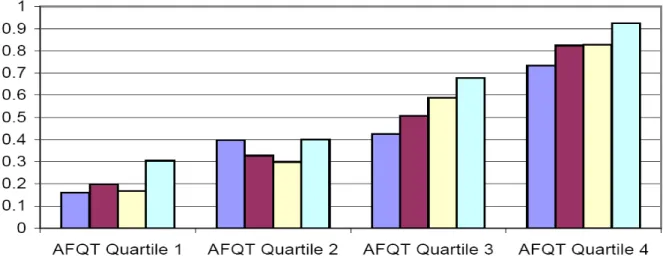
Documenti correlati
More than ten years ago, Ross Balzaretti published a highly interpretive article that discussed in partic- ular northern Italy’s economy between the eighth and the ninth centuries; 7
The main idea, in the application of elastic wavelets to the evolution of solitary waves, consists not only in the wavelet representation of the wave, but also in the assumption
We will relate the transmission matrix introduced earlier for conductance and shot noise to a new concept: the Green’s function of the system.. The Green’s functions represent the
4 Learn 20 objects with task A Present 20 items, one at a time, at strength ι word , while keeping the item-layer unit representing list A active at ι A and the day 1 unit active at
There is a consensus that elective laparoscopic sigmoid resection (for pro- cedures, see Appendix) may be an acceptable alternative to conventional sig- moid resection in patients
The important ophthalmologic signs and symptoms include nystagmus, ocular motor dysfunction, reduced visual acuity, visual field deficits, dyschromatopsia, an afferent pupillary
Abstract In this paper we analyze the effects of restricted participation in a two-period general equilibrium model with incomplete financial markets and two key elements:
149 In order to simultaneously encompass both bandwagon and snob effects, in the 150 updating preference functions we will introduce a threshold effect (see Granovetter 151 1978),