New insights into fish growth parameters estimation by means of length-based methods
Testo completo
Figura


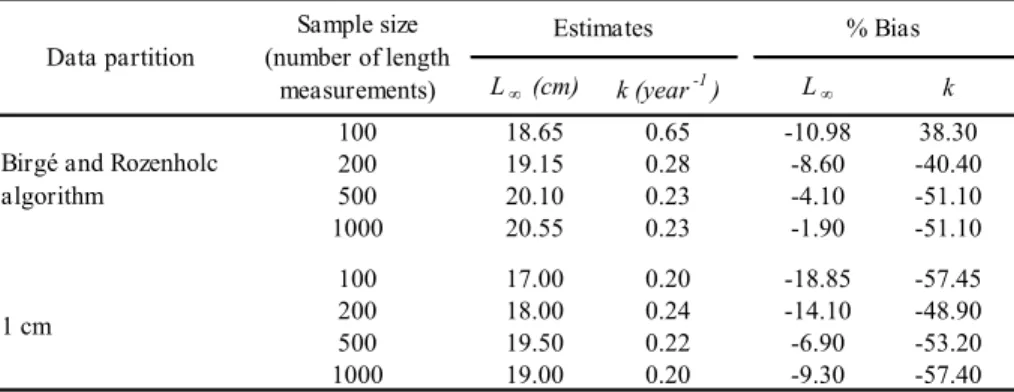
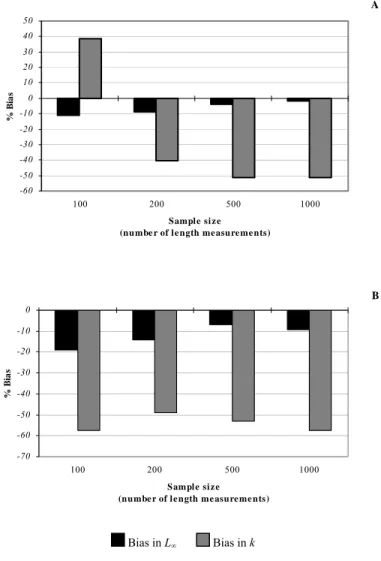
Documenti correlati
Heterocyclic analogs of benzanilide derivatives as potassium channel activators. 1,2,3-Triazol-carboxanilides and 1,2,3-(N-benzyl)-carboxamides as BK- potassium
A tutti quelli che hanno creduto in me senza caricarmi del peso delle loro aspettative e mi hanno sostenuto nei momenti
L’uso del paradigma analitico del morfotipo rurale ha consentito di descrivere e, soprattutto, di spazializzare, con una approssimazione riferibile alla scala
Al ritorno negli Stati Uniti, nel 1874, fu accolta con grande ammirazione sia a Boston che a Filadelfia, ricevendo applausi da molta parte della critica dell’epoca; Henry
Maximum interbuilding approaches corresponding to the un- controlled configuration (black line with squares), the active controller defined by the control gain matrix K (red line
Resta da stabilire, dunque, quali siano gli strumenti più efficaci per assicurare che effettivamente il ruolo dei consumatori all’interno del mercato non sia
The method presented in this article is based on three core algorithmic ideas: mean shift clustering to detect soma centers (Section 2.2), supervised semantic deconvolution by means
x the constitutive equations of both parent material and FSWed zone were used as input data in the FE simulation of the cold stamping process of the friction stir welded blanks;.